Understanding the AI Behind ChatGPT: How It Works
Large Language Models (LLMs), like OpenAI’s ChatGPT, represent a revolution in artificial intelligence. These systems are built on advanced neural networks, such as transformers, trained on massive datasets to predict, generate, and understand human language. While they have transformed industries, their development involves significant computational, financial, and environmental costs.
Summary
- Large Language Models (LLMs) like ChatGPT are transforming technology and communication.
- LLMs are mathematical representations of language, trained on vast datasets.
- Predictive text and smart assistants have long used early language models.
- The 1951 n-gram method, introduced by Claude Shannon, was an early attempt at modeling language.
- Neural networks enhanced these models by learning connections between words.
- The introduction of transformers in 2017 allowed parallel processing of text, speeding up training and boosting capabilities.
- Modern LLMs like ChatGPT, Meta’s Llama, and Google’s Gemini are trained on trillions of words, requiring over 100 billion parameters.
- Reinforcement learning with human feedback fine-tunes LLM outputs.
- Challenges include high costs and environmental concerns related to AI training.
- Despite challenges, LLMs continue to shape the future, influencing industries like healthcare, customer service, and education.
The Evolution of Language Models
Language models are mathematical systems designed to predict the likelihood of a sequence of words. For example, a language model would assign a high probability to a sentence like “The dog barked loudly” and a low probability to an illogical sequence like “Loudly barked dog the.”
Early Innovations: The N-Gram Method
The foundation of language models dates back to 1951, when Claude Shannon, a researcher at IBM, developed the n-gram approach. This method estimates the probability of sequences of words based on existing text. For example, the n-gram “black cat” is more likely than “cat quantum.”
However, longer n-grams presented challenges. Calculating probabilities for four-word sequences, for instance, proved exponentially harder than for two-word sequences. These limitations made early models inadequate for capturing connections between words far apart.
Explore more about Claude Shannon’s groundbreaking work on n-grams here.
Neural Networks: A Turning Point
To address n-gram limitations, researchers developed neural networks, which mimic how the human brain processes information. Unlike n-grams, neural networks can recognize relationships between words separated by long distances in a sentence.
Training these networks involves exposing them to large datasets, enabling them to predict the next word in a sequence. However, early neural networks faced challenges:
- Sequential Learning: Words had to be processed one at a time, slowing down training.
- Limited Context: Connections between distant words were weaker in practice.
Despite these hurdles, neural networks became a foundation for predictive text tools like those found in smartphones.
Discover more about neural networks’ influence here.
Transformers: Revolutionizing AI
In 2017, the introduction of transformers marked a turning point in language modeling. Transformers differ from traditional neural networks in their ability to process entire sentences at once, enabling:
- Parallel Training: Transformers analyze words simultaneously, reducing training time.
- Scalability: They can handle massive datasets, making them ideal for large-scale applications.
This innovation paved the way for modern large language models (LLMs) like ChatGPT, trained on trillions of words using billions of parameters.
Explore how transformers work here.
The Rise of Generative AI
LLMs are called “large” not just because of their size but because they are trained on vast datasets, often exceeding a trillion words. For context, an average human would take over 7,600 years to read such a volume.
Reinforcement Learning: The Human Touch
LLMs like ChatGPT are fine-tuned using reinforcement learning with human feedback. Here’s how it works:
- Humans provide prompts, such as questions or instructions.
- The AI generates responses, which are evaluated by humans.
- Feedback is used to guide the AI’s future outputs.
To reduce costs, some feedback is generated using AI models themselves. However, this process still involves significant financial and environmental costs, with training budgets often exceeding hundreds of millions of dollars.
Learn more about reinforcement learning here.
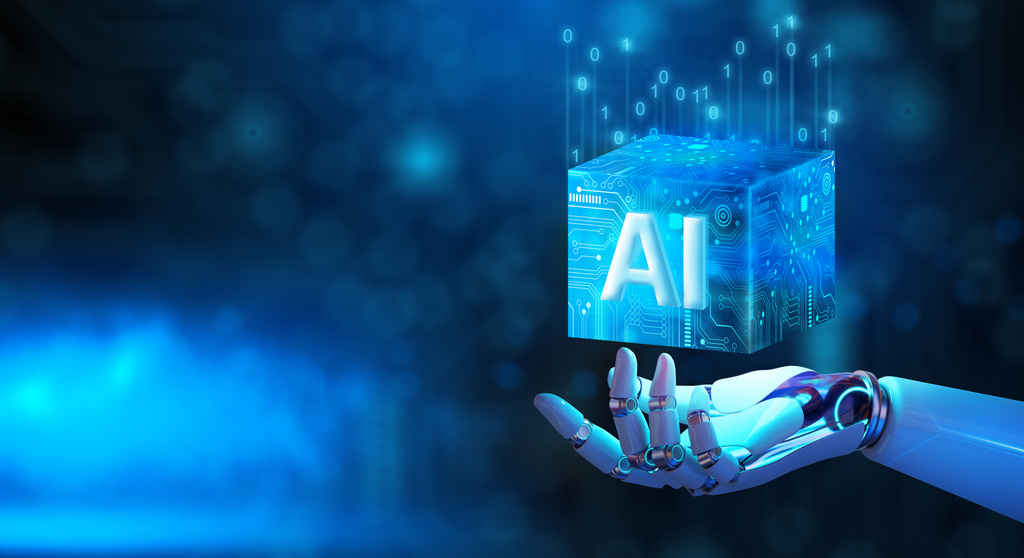
Applications of LLMs
LLMs like ChatGPT are reshaping industries:
Industry | Application |
---|---|
Healthcare | Assisting in diagnosis, summarizing medical research, and improving patient communication. |
Customer Service | Automating responses, resolving queries, and enhancing user experience. |
Education | Supporting personalized learning, answering questions, and simplifying complex topics. |
Modern LLMs have also enabled tools like ChatGPT, Meta’s Llama, and Google’s Gemini, which allow users to interact with AI using conversational prompts.
Explore Meta’s latest AI model, Llama, here.
Fun Fact: Environmental Impact of AI
Did you know? Training a single LLM can produce carbon dioxide emissions equivalent to five transatlantic flights. As AI adoption grows, addressing its environmental impact will be crucial.
Learn about sustainable AI initiatives here.
Challenges and Ethical Considerations
While LLMs have many benefits, they are not without challenges:
Challenge | Explanation |
---|---|
Bias in Training Data | Models may replicate biases present in the datasets they are trained on, leading to unfair or inaccurate responses. |
High Costs | Developing state-of-the-art LLMs requires significant financial investment, with training costs reaching hundreds of millions of dollars. |
Environmental Concerns | The computational power required for training leads to high energy consumption and carbon emissions. |
Misinformation Risks | LLMs can generate plausible but incorrect information, making them susceptible to misuse. |
Explore ethical concerns surrounding AI here.
Future Prospects of LLMs
Despite challenges, the future of LLMs is bright:
- Improved Accuracy: Research focuses on reducing biases and increasing factual accuracy.
- Sustainability: Efforts are underway to minimize environmental impact through energy-efficient training methods.
- Integration: LLMs are increasingly integrated into everyday tools, from search engines to virtual assistants.
Learn more about advancements in LLMs here.
References
- Large Language Models: How the AI Behind ChatGPT Works
- Claude Shannon’s Contributions to Information Theory
- Understanding Transformers in AI
- Meta’s AI Model: Llama
- Reinforcement Learning Explained